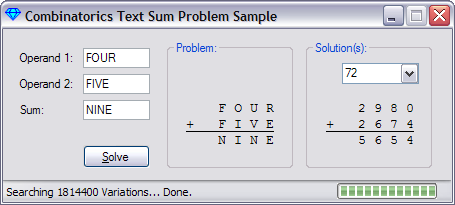
Introduction
Combinatorics has many applications within computer science for solving complex problems. However, it is under-represented in libraries since there is little application of Combinatorics in business applications. Fortunately, the science behind it has been studied by mathematicians for centuries, and is well understood and well documented. However, mathematicians are focused on how many elements will exist within a Combinatorics problem, and have little interest in actually going through the work of creating those lists. Enter computer science to actually construct these massive collections.
The C++ Standard Template Library (STL) brought us the very useful algorithm next_permutation
, which generates permutations of lists using iterators. However, most languages, including C#, do not have built in libraries for the generation of these lists. To fill this gap, a lot of work has been done in different languages focused on permutations, including a host of articles at the CodeProject. Even though so much quality work has already been done, I still found myself wanting for additional capabilities. In particular, a single solution that fulfils all of the following self-imposed requirements:
- Implemented with C# Generics
- Ability to count collection sizes before use
- Full support for Variations
- Object Oriented in implementation (that is, not a single function interface)
- Ease of use (e.g., works efficiently with
foreach
) - Consistent interface across combinatorial classes
- Fully documented, including Variations
- Support for both Repetitive and Non-Repetitive versions
If you're new to Combinatorics, then many of these requirements may not make sense. The Background section below includes a complete overview of the combinatorial concepts, including samples and how to calculate the size of the output sets. The Using the Code section follows up with the classes provided for each collection, and describes the features provided with these classes; this section contains everything you need to know to use the enclosed classes. Next, the Algorithm and Performance section discusses some of the options for implementation that were considered, and explains some of the major design decisions. Finally, the Sample section explains the small sample application included to demonstrate the use and need for Variations.
There are two common combinatorial concepts that are taught in every probability course. These are permutations and combinations. There is a lesser known collection known as a variation, which adapts features from both permutations and combinations. In addition, there are variants of each of these three which involve introducing repetition to the input or the output. These collections with repetition are also typically glossed over in introductory courses. So, the complete list of combinatorial collections is:
- Permutations
- Permutations with Repetition
- Combinations
- Combinations with Repetition
- Variations
- Variations with Repetition
Permutations deal with the ordering of a set of items, for example, how many ways a deck of 52 cards can be shuffled. Combinations deal subsets of a set of items, for example, how many 5 card poker hands can be dealt from a deck of 52 cards. In both cases, each card in the deck or in the hand is unique, so repetition is not a factor. However, problems do arise where repetition does occur in the input and/or output. For these cases, the repetition versions allow us more options in constructing our output sets. Variations are used when not only the subset provided by combinations is relevant but also the ordering within that subset. Each of these is covered below.
Permutations
Permutations are all possible orderings of a given input set. Each ordering of the input is called a permutation. When each item in the input set is different, there is only one way to generate the permutations. However, when two or more items in the set are the same, two different permutation sets are possible. These are called Permutations and Permutations with Repetition.
Permutations (i.e., without Repetition)
Standard permutations simply provide every single ordering of the input set:
Permutations of {A B C}:
{A B C}, {A C B}, {B A C}, {B C A}, {C A B}, {C B A}
The number of Permutations can be easily shown [2] to be P(n) = n!, where n is the number of items. In the above example, the input set contains 3 items, and the size is 3! = 6. This means that the number of permutations grows exponentially with n. Even a small n can create massive numbers of Permutations; for example, the number of ways to randomly shuffle a deck of cards is 52! or approximately 8.1E67.
Permutations with Repetition
Permutations with Repetition sets give allowance for repetitive items in the input set that reduce the number of permutations:
Permutations with Repetition of the set {A A B}:
{A A B}, {A B A}, {B A A}
The number of Permutations with Repetition is not as large, being reduced by the number and count of repetitive items in the input set. For each set of m identical items, the overall count is reduced by m!. In the above example, the input set contains 3 items with one subset of 2 identical items, the count is 3! / 2! = 6 / 2 = 3. The idea behind the count is easier than the formula since the formula requires the product of each repetitive set of size ri. The total size is Pr(n) = n! / Π(ri!) (where Π is the product operator). All of the collating and calculating is handled for us using the Permutation.Count
property.
The code library accompanying this article will determine, based on the input set, which type of permutation to use. Alternately, a type may be supplied to determine which permutation type to use.
Combinations
Combinations are subsets of a given size taken from a given input set. The size of the set is known as the Upper Index (n) and the size of the subset is known as the Lower Index (k). When counting the number of combinations, the terminology is generally "n choose k", and is known as the Binomial Coefficient [3]. Unlike permutations, combinations do not have any order in the output set. Like permutations, they do have two generation methods based on the repeating of output items. These are called Combinations and Combinations with Repetition.
Combinations (i.e., without Repetition)
Combinations can be thought of as throwing a set of n dominos into a hat and then retrieving k of them. Each domino can only be chosen once, and the order that they were fished out of the hat is irrelevant.
In a similar fashion, (n = 100) Scrabble tiles can be thrown into a bag, and the first player will select (k = 7) tiles. However, there are 9 A's in the bag, and selecting {A A A A A A A} would be a valid, although unlikely, draw. Since there are only 6 Ns in the bag, it is not possible to draw 7 Ns. As such, the values of the tiles are ignored for the Combinations, this differs from Permutations.
Combinations of {A B C D} choose 2:
{A B}, {A C}, {A D}, {B C}, {B D}, {C D}
The number of outputs in this particular example is the Binomial Coefficient. It is calculated as n! / ( k! * (n - k)! ) [4]. The Scrabble example above would give us 100! / (7! * 93!) = 16,007,560,800. Note that the answer to 100! is much larger than the answer as most of its magnitude was cancelled out by 93!.
Combinations with Repetition
Combinations with Repetition are determined by looking at a set of items, and selecting a subset while allowing repetition. For example, choose a tile from the scrabble bag above, write down the letter, and return the letter to the bag. Perform this 7 times to generate a sample. In this case, you could "draw" 7 Ns, just with a lower probability than drawing 7 As.
As such, Combinations with Repetition are a superset of Combinations, as seen in the following example:
Combinations with Repetition of {A B C D} choose 2:
{A A}, {A B}, {A C}, {A D}, {B B}, {B C}, {B D}, {C C}, {C D}, {D D}
Combinations are used in a large number of game type problems. For example, a deck of (n = 52) cards of which a (k = 5) card hand is drawn. Using the set of all combinations would allow for a brute force mechanism of solving statistical questions about poker hands.
Variations
Variations combine features of combinations and permutations, they are the set of all ordered combinations of items to make up a subset. Like combinations, the size of the set is known as the Upper Index (n) and the size of the subset is known as the Lower Index (k). And, the generation of variations can be based on the repeating of output items. These are called Variations and Variations with Repetition.
Variations (i.e., without Repetition)
Variations are permutations of combinations. That is, a variation of a set of n items choose k, is the ordered subsets of size k. For example:
Variations of {A B C} choose 2:
{A B}, {A C}, {B A}, {B C}, {C A}, {C B}
The number of outputs in this particular example is similar to the number of combinations of n choose k divided by the permutations of k. It can be calculated as V(n, k) = C(n, k) * P(k) = (n! / ( k! * (n - k)! )) * k! = n! / (n - k)!. The sample project included uses variations to select digits to be substituted for letters in a simple cryptographic word problem.
Variations with Repetition
Variations with Repetition expands on the set of variations, and allows items to be reused. Since each item can be re-used, this allows for variations to include all items in the output to be a single item from the input. For example:
Variations with Repetition of {A B C} choose 2:
{A A}, {A B}, {A C}, {B A}, {B B}, {B C}, {C A}, {C B}, {C C}
The size of the output set for variations is easier to compute since factorials are not involved. Each of the p positions can be filled from any of the n positions in the input set. The first item is one of n items, the second is also one of n, and the pth is also one of n. This gives us Vr(n, k) = nk total variations of n items choose k.
Enumerating the Sets
There are three class entry points in the code library, Permutations
, Combinations
, and Variations
. Each of these is a generic class based on the type T
of the items in the set. Each of these also generates a collection of collections based on the input set, making each a meta-collection. For ease of use, the classes implement IEnumerable<T>
, which returns an IList<T>
. However, this generic code is designed to make the consumption of each class easy. For example, using Permutations
:
char[] inputSet = {'A', 'B', 'C'};
Permutations<char> permutations = new Permutations<char>(inputSet);
foreach(IList<char> p in permutations) {
Console.WriteLine(String.Format("{{{0} {1} {2}}}", p[0], p[1], p[2]));
}
will generate:
{A B C}
{A C B}
{B A C}
{B C A}
{C A B}
{C B A}
Using Combinations
and Variations
is similar, but the Lower Index must also be specified. (The Upper Index is derived from the size of the input set.) For example:
char[] inputSet = { 'A', 'B', 'C', 'D' };
Combinations<char> combinations = new Combinations<char>(inputSet, 3);
string cformat = "Combinations of {{A B C D}} choose 3: size = {0}";
Console.WriteLine(String.Format(cformat, combinations.Count));
foreach(IList<char> c in combinations) {
Console.WriteLine(String.Format("{{{0} {1} {2}}}", c[0], c[1], c[2]));
}
Variations<char> variations= new Variations<char>(inputSet, 2);
string vformat = "Variations of {{A B C D}} choose 2: size = {0}";
Console.WriteLine(String.Format(vformat, variations.Count));
foreach(IList<char> v in variations) {
Console.WriteLine(String.Format("{{{0} {1}}}", v[0], v[1]));
}
will generate:
Combinations of {A B C D} choose 3: size = 4
{A B C}
{A B D}
{A C D}
{B C D}
Variations of {A B C D} choose 2: size = 12
{A B}
{A C}
{A D}
{B A}
{C A}
{D A}
{B C}
{B D}
{C B}
{D B}
{C D}
{D C}
With and Without Repetition
By default, Permutations
, Combinations
, and Variations
will generate the standard or no-repetition sets. Each class has an overloaded constructor that takes a GenerateOption
, which can either be GenerateOption.WithoutRepetition
(the default) or GenerateOption.WithRepetition
. For example, to generate a permutation set with and without repetition:
char[] inputSet = { 'A', 'A', 'C' };
Permutations<char> P1 = new Permutations<char>(inputSet,
GenerateOption.WithoutRepetition);
string format1 = "Permutations of {{A A C}} without repetition; size = {0}";
Console.WriteLine(String.Format(format1, P1.Count));
foreach(IList<char> p in P1) {
Console.WriteLine(String.Format("{{{0} {1} {2}}}", p[0], p[1], p[2]));
}
Permutations<char> P2 = new Permutations<char>(inputSet,
GenerateOption.WithRepetition);
string format2 = "Permutations of {{A A C}} with Repetition; size = {0}";
Console.WriteLine(String.Format(format2, P2.Count));
foreach(IList<char> p in P2) {
Console.WriteLine(String.Format("{{{0} {1} {2}}}", p[0], p[1], p[2]));
}
will generate:
Permutations of {A A C} without Repetition; size = 3
{A A C}
{A C A}
{C A A}
Permutations of {A A C} with Repetition; size = 6
{A A C}
{A C A}
{A A C}
{A C A}
{C A A}
{C A A}
Note that the input set for Permutations
must have repetition in it in order to see a difference in the output. Combinations
and Variations
will generate additional sets regardless of the similarity of incoming values.
Counting and Other Properties
While the intent of these classes is not to calculate Binomial Coefficients, each class does have a Count
property. This property will calculate the actual count of collections returned, without iterating through them. This is done by applying the formulas in the general discussion above and returning the value as a long
. Finally, the counting is done without internal overflow, which is important since 21! will overflow a long
.
The constructor parameters are also available; the upper index and lower index are available using UpperIndex
and LowerIndex
, respectively. The generator option is available through the Type
property. For example:
char[] alphanumeric = "ABCDEFGHIJKLMNOPQRSTUVWXYZ0123456789".ToCharArray();
Combinations<char> C = new Combinations<char>(alphanumeric, 10);
Console.WriteLine(String.Format("{0} choose {1} = {2}", C.UpperIndex,
C.LowerIndex, C.Count));
will generate:
36 choose 10 = 254186856
Finally, these common features are formalized through the IMetaCollection
interface that each of these classes implement.
At this point, we've covered everything that is required to understand and use these classes. The remainder of this discussion describes a bit of the background and decision making processes used in its implementation.
The numbers of permutations, combinations, and variations all grow exponentially. As such, a meta-collection enumerator on any but a trivial set of items will quickly exceed any available computation time. For example, a system that can enumerate a permutation of 10 items in 1 second will take over 1000 years to enumerate a permutation of 20 items. Since the performance for even the best algorithm will degrade, pretty much any algorithm will do. However, no developer could hold their head high without evaluating options and choosing the best algorithm for the job, even if it only knocks 50 years off a 1000 year run.
Choice of Algorithm
The ability to calculate a permutation is core to all of the combinatorial classes. Several algorithms have been developed for calculating permutations, three of which were evaluated for this implementation, namely Recursive, Lexicographic, and Heap's algorithms [1]. The Lexicographic algorithm [3] is perfectly suited for the IEnumerable
interface, since it uses the same GetNext()
style and requires very little adaptation. Both Recursive and Heap's algorithms are more efficient at generating permutations of integers, but need to be adapted for an IEnumerable
interface by either converting to an iterative algorithm or using C# continuation.
The first attempt was to take the more efficient heap based algorithms and un-roll them into iterative algorithms, and then to make them conform to the one result per call behavior of the IEnumerable
interface. Both heap algorithms stored quite a bit of state information and did not un-roll easily. Once done, they each went from about twice as fast as lexicographic to about twice as slow.
The second attempt was to use the continuation feature added to C# in .NET 2.0. This feature provides the yield return
syntax for quickly creating enumerators. It is also capable, with a bit of extra work, to handle recursive enumerators. The good news is that this mechanism works, but the performance was even worse than un-rolling the recursive algorithm by a factor of 4.
The lexicographic algorithm was therefore chosen as the best algorithm for this implementation.
Comparison Efficiency
The next issue revolved around performance of comparisons. All algorithms tested had to be changed to accommodate non-integer data. The lexicographic algorithm needs to compare objects to determine their sort order to be able to create a unique lexicographic order. The standard way of resolving this involves the IComparable
or IComparer
interfaces to determine the order. As this is a generic collection, it is relatively straightforward to adapt the integer comparison to an IComparer
provided comparison. Unfortunately, this comparison is called a lot and any inefficiency is magnified. Since this is a generic type comparison, the CLR does not optimize this nearly as efficiently as a value type.
The other problem with the use of a direct comparison on objects to permute is that the permutations will always be Repetitive instead of Non-Repetitive. That is, you cannot create a Non-Repetitive permutation of {A A B}
. Together with the above performance issues, another solution was required.
The final solution was to have a parallel array of integers on which the comparisons are performed. Both arrays will have items swapped in parallel, creating the correct output. The performance improves to within a few percent of the integer only solution that the algorithm started with. And the repetitive and non-repetitive set solutions are done by having different integer assignments in the parallel array. For repetitive, the assignment for {A A B}
is {1 2 3}
, and for non-repetitive, the assignment is {1 1 2}
. The IComparer
is used to sort the list, and then once more to check for neighboring duplicates if and only if the repetitive mode is chosen, but is not used during the GetNext()
call.
Combinations and Variations Efficiency
Most of the research around algorithms appear to have been focused on permutations, with less work being done on combinations and variations. As such, the combination and variation implementations use internal permutations to calculate their sets. For example, the Combinations
class uses a Permutations<bool>
class to indicate the positions to be included. The permutations of this underlying class indicate the subset to be selected by the combinations. Variations also use a similar mechanism, except for variations with repetition.
Therefore, the work on making permutations work efficiently is inherited by combinations and variations. Additional performance improvements in the combinations and variations implementations were not sought.
Counting
First, these classes are not designed to efficiently calculate tables of Binomial Coefficients. However, they do need to provide the Count of the collection that they are currently enumerating. As discussed above, these values can get really big, and can easily overflow 64 bit integers. But, the divisors in the count formulas bring the values back down to a manageable level. So, to properly count these collections, some type of large-integer calculations need to be performed.
The outputs of these counts are obviously always integers, so the prime factors of the denominators of the formulas will always be found in the prime factors of the numerators. Rather than performing large integer computations, a list of numerator prime factors and a list of denominator prime factors are calculated. Then, the list of denominators is removed from the list of numerators, and the product of the remaining numerators is returned. No attempt was made to compare the efficiency of this process, see paragraph above.
If you troll the code, you will find a SmallPrimeUtility
class that is used for the above algorithm. I make no warranty of the suitability of this class for anything else, it is a down and dirty class with no elegance to it at all. It is the Hyundai Excel of classes, it gets you where you're going, but not much else.
Want to See Some Real Performance
The classes created here are designed to exhaustively enumerate massive numbers of collections. For all but the most trivial sets, the amount of time required to enumerate exceeds all available computing capacity. So, there are times when it is necessary to brute force your way through a problem, but the fact that you can't for large N is what makes Computer Science fun. For small problems, this set of classes is suitable; for larger problems, this set can assist in quickly validating other more interesting algorithms. For large problems, you need a keen mind and not a dumb computer.
For more complex problems related to permutations or combinations, the total solution space, S
, grows far too quickly for it to be feasible to evaluate every option. These classes are designed to enumerate every permutation in S
; however, many problems present a smaller feasible search space, F
. [5]. For example, in the following numeric substitution problem:
F O U R
+ F I V E
---------
N I N E
There are 8 variables {F O U R I V E N}
to be chosen from 10 digits {0 1 2 3 4 5 6 7 8 9}
. This implies that there are variations of 10 choose 8 possibilities in the solution space S
, which is 10! / 2! = 1,814,800 variations for the solution space S
. However, several observations can be made; for example, the fourth column provides us with R + E = E, which implies R = 0. This has simplified the problem to 7 unknown variables to be chosen from 9 digits, or 9! / 2! = 181,480 variations. Further, the first column implies that F <= 4, as we can't allow an overflow on this column. This removes 5/9th of the remaining variations that need to be tested, leaving 80,640 variations to test. Additional simplifications exist, and will reduce the space even further.
The point being that the more we know about the specifics of a problem, the more we are likely to be able to reduce the size of the feasible search space, the more likely we can solve the problem in a reasonable amount of time.
The FOUR + FIVE problem above is just one of a nearly inexhaustible supply of numeric substitution letter problems. This can be used to show that THREE + SEVEN = EIGHT, and even that WRONG + WRONG = RIGHT. Using the attached sample, you can enter the operands and the sum, and have the program search for any and all solutions. The program uses the Variations
class to exhaustively search every possible variation, and checks if the variation satisfies the equation.

User Interface
To compute a problem, simply enter the operands, e.g., "FOUR" and "FIVE", into the operands fields, and the sum, e.g. "NINE", into the sum field. The Problem frame will automatically update to present the summation problem. Please ensure that there are no more than 10 distinct characters entered, as no validation is done on this input. Entering more than 10 will ensure that no solution will be found. There are many more ways to ensure that no solution exists such as A + BC = DEFGH. The interface will happily accept these inputs and then return that no solutions were found.
Once the problem is entered, clicking Solve will enumerate all possible variations of the digits 1 through 10 for each character in the input. The status bar will display the total number of Variations that will be checked; this will vary based on the number of unique characters in the inputs. This will vary from a low of 90 variations to solve A + A = B, to a high of 3,628,800 when 10 characters are used such as in ABCDE + ABCDE = FGHIJ. The progress bar will indicate the overall progress, which should only take a few seconds for any of these problems. The Solution(s) frame will show the total number found and the most recently found solution, as the program progresses.
After all solutions have been found, select the drop down list in the Solution(s) frame to move through all of the solutions that were found.
Code
The Facet.Combinatorics
namespace is contained in the sample's Combinatorics sub-directory. It contains all of the code required to support Permutations
, Combinations
, and Variations
. It has no dependencies aside from the standard .NET 2.0 System
references. This directory can be lifted into other solutions to utilize these classes, no separate library is created.
The form code is plain vanilla, not production ready UI stuff which can be safely ignored. It has all of the UI elements, and defers the problem solving to the TextSumProblem
class which encapsulates this particular problem.
The meat of the sample is in the TextSumProblem
which creates every possible variation for the problem. The core of the solution is to generate all variations of integers that are possible candidates for the letters of the problem. In the sample, the Solve()
method has logic similar to:
int[] ints = {0, 1, 2, 3, 4, 5, 6, 7, 8, 9};
Variations<int> variations = new Variations<int>(ints, 8);
foreach(IList<int> variation in variations) {
if(Satisfies(variation) == true) {
}
}
Finally, the TextSumProblem
has a pair of events that signal when a candidate solution has been tried and also when a solution has been found. The form subscribes to these events to provide real time feedback of the problem's progress.
Any number of funny examples can be created to "prove" all sorts of contradictions. Most of them will have multiple solutions (ID + EGO = SELF has 1200 solutions, although it's a bit of a cheat), drop me a note if you find a good one that has a single unique solution, I've never been able to find one.
References
- A. Bogomolny, "Counting And Listing All Permutations", Interactive Mathematics Miscellany and Puzzles (2008-04-28)
- J. Claeys, "Counting Problems", MATH-abundance, (2008-04-28)
- E. W. Dijkstra, A Discipline of Programming, Prentice-Hall, 1997
- R. L. Graham, D. E. Knuth, O. Patashnik, Concrete Mathematics, Addison-Wesley, 1994
- Z. Michalewicz, D. B. Fogel, How to Solve It: Modern Heuristics, Springer-Verlag, 2000
History
- 2008-05-24
- Fixed spelling errors in article.
- Modified current method of the permutations iterator to return copy of list.
- 2008-05-14