Here we look at the Hopper environment which represents a single disembodied leg, training with SAC in the Hopper environment, and we train a simple four-legged creature to scuttle off at speed without falling over.
Introduction
In the previous article, we got set up with the Bullet physics simulator as a basis for doing some reinforcement learning in continuous control environments. The simplest way to get started was to use the cornerstone RL environment we were already familiar with from the earlier series of articles: the Cartpole.
However, PyBullet has a lot more environments to offer. For most of this series we shall be teaching a humanoid robot how to walk, but first it’s worth having a look at a couple of other environments: Hopper and Ant.
Hopper
The Hopper environment is quite fun: It represents a single disembodied leg. The objective is to train a policy that makes the leg hop away as fast as possible.
Let’s take a look at how complex this environment is.
>>> import gym
>>> import pybullet_envs
>>> env = gym.make('HopperBulletEnv-v0')
>>> env.observation_space
Box(15,)
>>> env.action_space
Box(3,)
Again we are in continuous spaces for both the observations and actions. This time, both are more complicated. The observation space has 15 dimensions instead of four for the CartPole, and the action space has three dimensions instead of just one.
Code
Here is the code I used for training with SAC in the Hopper environment.
import pyvirtualdisplay
_display = pyvirtualdisplay.Display(visible=False, size=(1400, 900))
_ = _display.start()
import ray
from ray import tune
from ray.rllib.agents.sac import SACTrainer
import pybullet_envs
ray.shutdown()
ray.init(include_webui=False, ignore_reinit_error=True)
ENV = 'HopperBulletEnv-v0'
import gym
from ray.tune.registry import register_env
def make_env(env_config):
import pybullet_envs
return gym.make(ENV)
register_env(ENV, make_env)
TARGET_REWARD = 6000
TRAINER = SACTrainer
tune.run(
TRAINER,
stop={"episode_reward_mean": TARGET_REWARD},
config={
"env": ENV,
"num_workers": 7,
"num_gpus": 1,
"monitor": True,
"evaluation_num_episodes": 50,
}
)
Graph
I killed the training process after 22.6 hours. Learning progress looked like this:
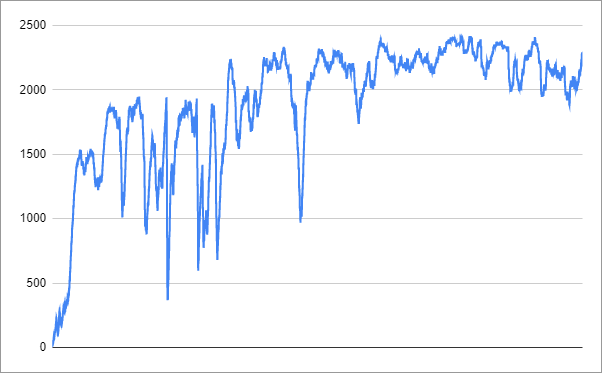
Video
The video from the last progress snapshot looked like this, showing that the agent had learned to hop along nicely.

Ant
Spiders have eight legs, insects have six, but PyBullet Ant agents have only four. In the Ant environment, we must train a simple four-legged creature to scuttle off at speed without falling over.
Again, let’s inspect the environment before we start training.
>>> import gym
>>> import pybullet_envs
>>> env = gym.make(AntBulletEnv-v0')
>>> env.observation_space
Box(28,)
>>> env.action_space
Box(8,)
This represents another step up in dimensionality: from 15 to 28 for the observation space and from three to eight in the action space. It’s not surprising that a four-legged ant should be more complex to train than a single leg.
Code
Because this is another simple environment, we can just change the environment specified in the code without having to tweak any of the learning parameters:
ENV = 'AntBulletEnv-v0'
Graph
Again I killed the process after 25.8 hours. You might prefer to set TARGET_REWARD to 3000.
Learning progress looked like this:

Video
Here is the video of the final snapshot. The agent makes fast progress, and there doesn’t seem to be much left for it to learn.

Next Time
The rest of the articles in this series focus on one particular, more complex environment that PyBullet makes available: Humanoid, in which we must train a human-like agent to walk on two legs. We start this in the next article, using the Proximal Policy Optimisation (PPO) algorithm.