You are climbing a staircase. It takes n steps to reach the top. Each time you can either take 1 or 2 steps. The goal is to find how many distinct steps we can make to get to the top of the stairs.
Ah dynamic programming. It strikes fear into the hearts of many computer science students and interviewers alike.They don’t appear often in interviews, you might be able to get away never needing to know them, but when they show up, it’ll be messy!
Luckily for us, dynamic programming like everything else in a coding interview, is just an algorithm. With enough practice, you’ll be able to get an intuition and solve DP problems in no time!
Dynamic programming is a clever technique that optimizes a brute force solution by solving for the smaller subproblems that lead to the answer. This approach of solving and reusing existing subproblems help us achieve the optimal solution.
To help understand DP, in this article, we will talk about the top down (memoization) strategy to solve a popular DP problem: Climbing Stairs. In another article, we will talk about how we can solve it with the bottom up strategy.
Problem Statement
You are climbing a staircase. It takes n steps to reach the top. Each time you can either take 1 or 2 steps.
The goal is to find how many distinct steps we can make to get to the top of the stairs.
Understanding the Problem
The idea around this problem is pretty straightforward. We want to count unique permutations of steps that will help us reach the nth step.
This picture will show us how many different steps we can take to reach n=4 from 0. In this instance, there are 5 unique combinations of steps that we can take to reach the 4th floor.
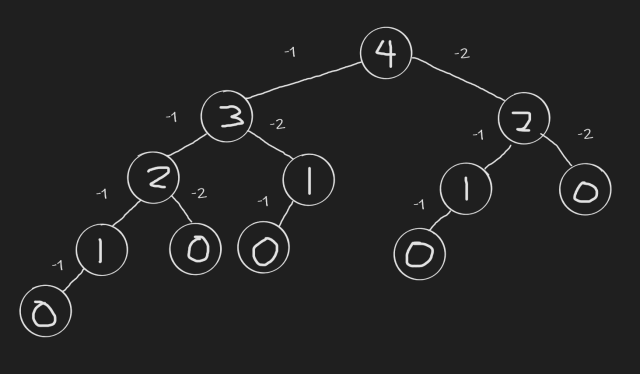
High Level Algorithm
DP is an optimization on top of sub-problems (usually recursion), so before we use it, we still need to solve the problem.
Looking at the picture of possibilities above, one way to find the solution is to try every combination of steps until we reach our nth step. In our case, we’re starting from n and counting down to 0 to simplify the problem.
For problems like these where we try every combination in a specific state (i.e., which step we’re on), we use recursion!
Just like in our article about Postorder Tree traversal with recursion, we need to define our base case and our recursive case.
Base case:
- n < 0: return 0, we went too far
- n == 0: return 1, we reached the nth step
Recursive case:
- N > 0: try taking 1 step and 2 step
Now you might be wondering, why is this a dynamic programming question? If you look back at the picture. You’ll notice that we’ve reached the same step multiple times in different ways.
When n=1:

Whenever we’re here, we always have one possible unique path.
When n=2:

Whenever we’re here, there will always be two possible unique paths.
Notice how we are repeating the same calculations over and over. For something small like n=4, it might not seem like a big deal, however for n=1000, we will have millions of millions of combinations (2^1000 to be specific) to calculate.
The idea behind DP is that instead of calculating the unique path to the same subproblem (like n=2), we should save the results and reuse it when we see it again.
For example: If n=2, there will always be 2 distinct ways to go to 0. We either take 2 steps or we take 2, 1 step.
Instead of doing this calculation over and over, if we save the result the first time we calculate n=2, we can save a lot of unnecessary calculations in the future.
In this picture, assuming we start by always taking 1 step and save the results, we can prevent calculating n=1 and n=2 when we see them a second time.

This is the top down strategy or memoization. We start at our goal, n=4 work our way down to our base case of n=0, and then bubble back up to the top storing all the calculations we have made.
Memoization is just a technique where we would cache or store the value of a certain state inside an array/map. For example, if we are on the 2nd step going down to 0, we will always have 2 ways of achieving it, so memo[2] = 2
For DP problems, it’s always important to think about everything as states or subproblems to cache the results in a data structure. In this problem, our state is the number of steps we have left to take.
The idea is that we will always first check to see if we have seen the state before. If we haven’t, we will do the calculation and then store the answer. If we have, we will just return our answer.
The general strategy to solve a DP problem by using top down is to:
- Solve the problem using a Brute force recursive solution.
- Create an array to store each state you encounter and cache your calculation in the recursive case.
- Add another base case where you reuse the cache value if you have seen the state.
The hard part of solving any DP problem is not actually the DP itself, it’s just being able to solve the problem with recursion.
Once you have the recursive solution, it’s just creating an array to cache your calculations.
Walking through an example, let’s say we want to calculate the number of unique paths for N=4.
We will start out with an empty cache of size N+1.

The first thing we will do is explore taking 1 step:

Nothing interesting happens and we continue taking 1 step until we reach our base case, n=0.

At N=0, we return 1 and we go back up to N=1.

At this point, we count the number of paths we can make by taking 2 steps, and we have made the conclusion that n=1 only has 1 unique path. We save this in our cache for index 1.

We then go back up to n=2 and calculate how many paths we can take if we take 2 steps. This would bring us to our base case of n=0 so we return 1.

Now that we have the unique path for each step on n=2, we know there are 2 unique paths we can take and we save it in our cache.

Continuing back up to n=3, if we explore taking 2 steps down the stairs, we would reach n=1, because of our cache, we know that would return 1 unique path. As a result, we don’t need to do the calculation again.

Using our cache, we can now calculate that n=3 has a total of 3 unique paths it can make. Once again, we’ll update our cache.

Finally, we return back to n=4. We take 2 steps from there and we reach n=2. Looking at our cache, we already know that it has 2 unique paths it generates. As a result, we don’t need to explore it.

With this, we now know that n=4 has 5 unique paths it can make, which is our answer.
Runtime and Space Complexity
Runtime
The brute force recursive solution has a runtime of O(2^N). This is because for every N step, we can make 2 decisions: take 1 step or take 2 steps. This might be okay if N is small, but it exponentially grows larger as N increases.
By utilizing DP, we bring this problem down to O(N), because we only need to calculate the value for each step once.
Space Complexity
The space complexity of both of our solutions is O(N). In the brute force recursive solution, we are recursively going from 0 to N keeping them in our call stack. Every time we finish a path, we would pop it out of our callstack so at most, it’ll be O(N).
For the DP solution, we are doing the same thing, and we are also storing the answers to each of our states in an array.
Implementation
Recursive solution:
class Solution {
public int climbStairs(int n) {
return helper(n);
}
private int helper(int n) {
if (n == 0) {
return 1;
} else if (n < 0) {
return 0;
} else {
return helper(n-1) + helper(n-2);
}
}
}
Memoization solution:
class Solution {
private int[] memo;
public int climbStairs(int n) {
memo = new int[n+1];
return helper(n);
}
private int helper(int n) {
if (n < 0) {
return 0;
} else if (memo[n] != 0) {
return memo[n];
} else if (n == 0) {
return 1;
} else {
memo[n] = helper(n-1) + helper(n-2);
return memo[n];
}
}
}
Conclusion
To solve Climbing Stairs, we need to be able to solve the problem recursively and then apply DP via memoization (caching) to help optimize the runtime.
Hopefully after going through this problem, you realized now that DP isn’t scary, it’s just an optimization on top of a brute force solution.
That’s the end for solving this problem with using memoization, in a future article, we will look at solving the same problem using another technique called the bottoms up approach where we will build up to the answer by building up from our base case.