Here we’ll show you how to implement a residual-based generator and train the resulting CycleGAN on a medical dataset.
Introduction
In this series of articles, we’ll present a Mobile Image-to-Image Translation system based on a Cycle-Consistent Adversarial Networks (CycleGAN). We’ll build a CycleGAN that can perform unpaired image-to-image translation, as well as show you some entertaining yet academically deep examples. We’ll also discuss how such a trained network, built with TensorFlow and Keras, can be converted to TensorFlow Lite and used as an app on mobile devices.
We assume that you are familiar with the concepts of Deep Learning, as well as with Jupyter Notebooks and TensorFlow. You are welcome to download the project code.
In the previous article of this series, we trained and evaluated a CycleGAN that used a U-Net-based generator. In this article, we’ll implement a CycleGAN with a residual-based generator.
CycleGAN from Scratch
The original CycleGan was first built using a residual-based generator. Let’s implement a CycleGAN of this type from scratch. We’ll build the network and train it to reduce artifacts in fundus images using a dataset of fundi with and without artifacts.
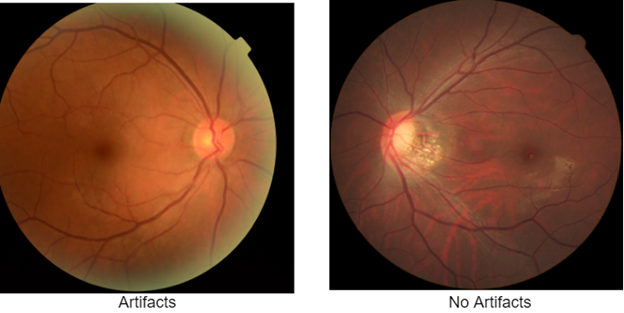
The network will translate fundus images with artifacts to those without artifacts and vice versa, as shown above.
The CycleGAN design will include the following steps:
- Building discriminators
- Building the residual block
- Building generators
- Building the complete model
Before we start loading our data, let’s import some necessary libraries and packages.
from random import random
from numpy import load
from numpy import zeros
from numpy import ones
from numpy import asarray
from numpy.random import randint
from keras.optimizers import Adam
from keras.initializers import RandomNormal
from keras.models import Model
from keras.models import Input
from keras.layers import Conv2D
from keras.layers import Conv2DTranspose
from keras.layers import LeakyReLU
from keras.layers import Activation
from keras.layers import Concatenate
from keras_contrib.layers.normalization.instancenormalization import InstanceNormalization
from matplotlib import pyplot
Loading Dataset
Contrary to what we’ve done in the previous article, this time we’ll use a local machine (instead of Google Colab) to train the CycleGAN. Hence, the fundus dataset should be first downloaded and processed. We’ll use Jupyter Notebook and TensorFlow to build and train this network.
from os import listdir
from numpy import asarray
from numpy import vstack
from keras.preprocessing.image import img_to_array
from keras.preprocessing.image import load_img
from numpy import savez_compressed
def load_images(path, size=(256,256)):
data_list = list()
for filename in listdir(path):
pixels = load_img(path + filename, target_size=size)
pixels = img_to_array(pixels)
data_list.append(pixels)
return asarray(data_list)
path = r'C:/Users/abdul/Desktop/ContentLab/P3/Fundus/'
dataA1 = load_images(path + 'trainA/')
dataAB = load_images(path + 'testA/')
dataA = vstack((dataA1, dataAB))
print('Loaded dataA: ', dataA.shape)
dataB1 = load_images(path + 'trainB/')
dataB2 = load_images(path + 'testB/')
dataB = vstack((dataB1, dataB2))
print('Loaded dataB: ', dataB.shape)
filename = 'Artifcats.npz'
savez_compressed(filename, dataA, dataB)
print('Saved dataset: ', filename)
Once the data is loaded, it’s time to create a function that displays some of the training images:
from numpy import load
from matplotlib import pyplot
data = load('Artifacts.npz')
dataA, dataB = data['arr_0'], data['arr_1']
print('Loaded: ', dataA.shape, dataB.shape)
n_samples = 3
for i in range(n_samples):
pyplot.subplot(2, n_samples, 1 + i)
pyplot.axis('off')
pyplot.imshow(dataA[i].astype('uint8'))
for i in range(n_samples):
pyplot.subplot(2, n_samples, 1 + n_samples + i)
pyplot.axis('off')
pyplot.imshow(dataB[i].astype('uint8'))
pyplot.show()

Building Discriminators
As we’ve discussed earlier, a discriminator is a CNN that consists of many convolutional layers, as well as LeakReLU and Instance Normalization layers.
def define_discriminator(image_shape):
init = RandomNormal(stddev=0.02)
in_image = Input(shape=image_shape)
d = Conv2D(64, (4,4), strides=(2,2), padding='same', kernel_initializer=init)(in_image)
d = LeakyReLU(alpha=0.2)(d)
d = Conv2D(128, (4,4), strides=(2,2), padding='same', kernel_initializer=init)(d)
d = InstanceNormalization(axis=-1)(d)
d = LeakyReLU(alpha=0.2)(d)
d = Conv2D(256, (4,4), strides=(2,2), padding='same', kernel_initializer=init)(d)
d = InstanceNormalization(axis=-1)(d)
d = LeakyReLU(alpha=0.2)(d)
d = Conv2D(512, (4,4), strides=(2,2), padding='same', kernel_initializer=init)(d)
d = InstanceNormalization(axis=-1)(d)
d = LeakyReLU(alpha=0.2)(d)
d = Conv2D(512, (4,4), padding='same', kernel_initializer=init)(d)
d = InstanceNormalization(axis=-1)(d)
d = LeakyReLU(alpha=0.2)(d)
patch_out = Conv2D(1, (4,4), padding='same', kernel_initializer=init)(d)
model = Model(in_image, patch_out)
model.compile(loss='mse', optimizer=Adam(lr=0.0002, beta_1=0.5), loss_weights=[0.5])
return model
Once the discriminator is built, we can create a copy of it so that we have two identical discriminators: DiscA
and DiscB
.
image_shape=(256,256,3)
DiscA=define_discriminator(image_shape)
DiscB=define_discriminator(image_shape)
DiscA.summary()
Building the Residual Block
The next step is to create the residual block for our generators. This block is a set of 2D convolutional layers, where every two layers are followed by an instance normalization layer.
def resnet_block(n_filters, input_layer):
init = RandomNormal(stddev=0.02)
g = Conv2D(n_filters, (3,3), padding='same', kernel_initializer=init)(input_layer)
g = InstanceNormalization(axis=-1)(g)
g = Activation('relu')(g)
g = Conv2D(n_filters, (3,3), padding='same', kernel_initializer=init)(g)
g = InstanceNormalization(axis=-1)(g)
g = Concatenate()([g, input_layer])
return g
Building the Generator
The residual block’s output will pass through the last part of the generator (the decoder), where an image will be upsampled and resized to its original size. Since the encoder is not defined yet, we’ll build a function that defines both the decoder and encoder parts and connects them to the residual block.
def define_generator(image_shape, n_resnet=9):
init = RandomNormal(stddev=0.02)
in_image = Input(shape=image_shape)
g = Conv2D(64, (7,7), padding='same', kernel_initializer=init)(in_image)
g = InstanceNormalization(axis=-1)(g)
g = Activation('relu')(g)
g = Conv2D(128, (3,3), strides=(2,2), padding='same', kernel_initializer=init)(g)
g = InstanceNormalization(axis=-1)(g)
g = Activation('relu')(g)
g = Conv2D(256, (3,3), strides=(2,2), padding='same', kernel_initializer=init)(g)
g = InstanceNormalization(axis=-1)(g)
g = Activation('relu')(g)
for _ in range(n_resnet):
g = resnet_block(256, g)
g = Conv2DTranspose(128, (3,3), strides=(2,2), padding='same', kernel_initializer=init)(g)
g = InstanceNormalization(axis=-1)(g)
g = Activation('relu')(g)
g = Conv2DTranspose(64, (3,3), strides=(2,2), padding='same', kernel_initializer=init)(g)
g = InstanceNormalization(axis=-1)(g)
g = Activation('relu')(g)
g = Conv2D(3, (7,7), padding='same', kernel_initializer=init)(g)
g = InstanceNormalization(axis=-1)(g)
out_image = Activation('tanh')(g)
model = Model(in_image, out_image)
return model
And now, we define the generators genA
and genB
.
genA=define_generator(image_shape, 9)
genB=define_generator(image_shape, 9)
Building CycleGAN
With the generators and discriminators defined, we can now build the entire CycleGAN model and set its optimizers and other learning parameters.
def define_composite_model(g_model_1, d_model, g_model_2, image_shape):
g_model_1.trainable = True
d_model.trainable = False
g_model_2.trainable = False
input_gen = Input(shape=image_shape)
gen1_out = g_model_1(input_gen)
output_d = d_model(gen1_out)
input_id = Input(shape=image_shape)
output_id = g_model_1(input_id)
output_f = g_model_2(gen1_out)
gen2_out = g_model_2(input_id)
output_b = g_model_1(gen2_out)
model = Model([input_gen, input_id], [output_d, output_id, output_f, output_b])
opt = Adam(lr=0.0002, beta_1=0.5)
model.compile(loss=['mse', 'mae', 'mae', 'mae'], loss_weights=[1, 5, 10, 10], optimizer=opt)
return model
Now let’s define two models (A and B), where one will translate fundus images artifacts to no-artifact fundi (AtoB
), and the other one will translate no-artifacts to artifact fundus images (BtoA
).
comb_modelA=define_composite_model(genA,DiscA,genB,image_shape)
comb_modelB=define_composite_model(genB,DiscB,genA,image_shape)
Training CycleGAN
Now that our model is complete, we’ll create a training function that defines the training parameters and calculates the generator and discriminators losses in addition to updating the weights during training. This function will operate as follows:
- Pass an image to the generators.
- Get a generated image by generators.
- Pass the generated images back to the generators to verify that we can predict the original image from the generated image.
- Using the generators, perform identity mapping of the real images.
- Pass the images generated in step 1 to the corresponding discriminators.
- Find the generators’ total loss (adversarial + cycle + identity).
- Find the discriminators’ loss.
- Update generator weights.
- Update discriminator weights.
- Return losses in a dictionary.
def train(d_model_A, d_model_B, g_model_AtoB, g_model_BtoA, c_model_AtoB, c_model_BtoA, dataset):
n_epochs, n_batch, = 30, 1
n_patch = d_model_A.output_shape[1]
trainA, trainB = dataset
poolA, poolB = list(), list()
bat_per_epo = int(len(trainA) / n_batch)
n_steps = bat_per_epo * n_epochs
for i in range(n_steps):
X_realA, y_realA = generate_real_samples(trainA, n_batch, n_patch)
X_realB, y_realB = generate_real_samples(trainB, n_batch, n_patch)
X_fakeA, y_fakeA = generate_fake_samples(g_model_BtoA, X_realB, n_patch)
X_fakeB, y_fakeB = generate_fake_samples(g_model_AtoB, X_realA, n_patch)
X_fakeA = update_image_pool(poolA, X_fakeA)
X_fakeB = update_image_pool(poolB, X_fakeB)
g_loss2, _, _, _, _ = c_model_BtoA.train_on_batch([X_realB, X_realA], [y_realA, X_realA, X_realB, X_realA])
dA_loss1 = d_model_A.train_on_batch(X_realA, y_realA)
dA_loss2 = d_model_A.train_on_batch(X_fakeA, y_fakeA)
g_loss1, _, _, _, _ = c_model_AtoB.train_on_batch([X_realA, X_realB], [y_realB, X_realB, X_realA, X_realB])
dB_loss1 = d_model_B.train_on_batch(X_realB, y_realB)
dB_loss2 = d_model_B.train_on_batch(X_fakeB, y_fakeB)
print('>%d, dA[%.3f,%.3f] dB[%.3f,%.3f] g[%.3f,%.3f]' % (i+1, dA_loss1,dA_loss2, dB_loss1,dB_loss2, g_loss1,g_loss2))
if (i+1) % (bat_per_epo * 1) == 0:
summarize_performance(i, g_model_AtoB, trainA, 'AtoB')
summarize_performance(i, g_model_BtoA, trainB, 'BtoA')
if (i+1) % (bat_per_epo * 5) == 0:
save_models(i, g_model_AtoB, g_model_BtoA)
Here are some functions that will be used during training.
def load_real_samples(filename):
data = load(filename)
X1, X2 = data['arr_0'], data['arr_1']
X1 = (X1 - 127.5) / 127.5
X2 = (X2 - 127.5) / 127.5
return [X1, X2]
def generate_real_samples(dataset, n_samples, patch_shape):
ix = randint(0, dataset.shape[0], n_samples)
X = dataset[ix]
y = ones((n_samples, patch_shape, patch_shape, 1))
return X, y
def generate_fake_samples(g_model, dataset, patch_shape):
X = g_model.predict(dataset)
y = zeros((len(X), patch_shape, patch_shape, 1))
return X, y
def update_image_pool(pool, images, max_size=50):
selected = list()
for image in images:
if len(pool) < max_size:
pool.append(image)
selected.append(image)
elif random() < 0.5:
selected.append(image)
else:
ix = randint(0, len(pool))
selected.append(pool[ix])
pool[ix] = image
return asarray(selected)
We add some more functions to save the best models and visualize the performance of the artifact reduction in the fundus images.
def save_models(step, g_model_AtoB, g_model_BtoA):
filename1 = 'g_model_AtoB_%06d.h5' % (step+1)
g_model_AtoB.save(filename1)
filename2 = 'g_model_BtoA_%06d.h5' % (step+1)
g_model_BtoA.save(filename2)
print('>Saved: %s and %s' % (filename1, filename2))
def summarize_performance(step, g_model, trainX, name, n_samples=5):
X_in, _ = generate_real_samples(trainX, n_samples, 0)
X_out, _ = generate_fake_samples(g_model, X_in, 0)
X_in = (X_in + 1) / 2.0
X_out = (X_out + 1) / 2.0
for i in range(n_samples):
pyplot.subplot(2, n_samples, 1 + i)
pyplot.axis('off')
pyplot.imshow(X_in[i])
for i in range(n_samples):
pyplot.subplot(2, n_samples, 1 + n_samples + i)
pyplot.axis('off')
pyplot.imshow(X_out[i])
filename1 = '%s_generated_plot_%06d.png' % (name, (step+1))
pyplot.savefig(filename1)
pyplot.close()
train(DiscA, DiscB, genA, genB, comb_modelA, comb_modelB, dataset)
Evaluating Performance
Using the above functions, we trained the network for 30 epochs. The results show that our network was capable of reducing the artifacts in fundus images.
Results of artifact to no-artifact translations (AtoB) are shown below:

The no-artifacts to artifacts (BtoA
) fundus image translations are also computed; here are some examples.

Conclusion
As AI Pioneer Yann LeCun said about GAN, "(It is) the most interesting idea in deep learning in the last 10 years". We hope that, through this series, we’ve helped you to understand why GANs are some very interesting ideas. We are aware that you may have found the concepts presented in the series a bit heavy and ambiguous, but that it is totally fine. CycleGAN is incredibly difficult to grasp in one read and it’s all right to go over the series a few times before you get it.
Lastly, if you liked what you encountered in this series, always remember that you can improve upon it! Why not take your new skills, create something great, then write about it and share it on CodeProject?