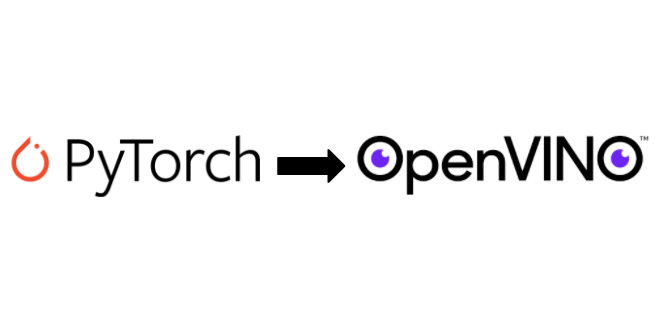
Note: This article was created with OpenVINO 2022.1. If you want to know how to use the old API of OpenVINO 2021.4 please check this notebook.
Although PyTorch is a great framework for AI training and can be used for inference, OpenVINO™ Toolkit can provide additional benefits in case of inference performance as it’s heavily optimized for this task. To use it, you need just 3 simple steps: install OpenVINO, convert and optimize your model and run the inference. To show you the whole process we decided to use the FastSeg model, a network for semantic segmentation, pre-trained on the Cityscapes dataset.
OpenVINO is able to run the inference for networks in Intermediate Representation (IR) format. So, you must convert your network with Model Optimizer, a command-line tool from the Development Package. The simplest method to install it is using PyPi:
pip install openvino-dev[pytorch,onnx]
The first step is to export the model to ONNX format. You need to use Opset version 11 as that version is supported by OpenVINO. Also, for this particular model, constant folding is not allowed.
from fastseg import MobileV3Large
model = MobileV3Large.from_pretrained().cpu().eval()
dummy_input = torch.randn(1, 3, 512, 1024)
torch.onnx.export(model, dummy_input, "fastseg1024.onnx", opset_version=11, do_constant_folding=False)
OpenVINO supports ONNX directly, so you can load the exported model to the runtime and start the processing. However, to get an even better performance it’s desirable to convert and optimize the model to IR. Use the following command in the terminal:
mo --input_model fastseg1024.onnx --input_shape "[1,3,512,1024]"
It implies you’re converting model.onnx with input size equals 1024x512x3 (W x H x C). Of course, you may provide other parameters such as pre-processing or model precision (FP32 or FP16):
mo --input_model fastseg1024.onnx --input_shape "[1,3,512,1024]" --mean_values="[123.675,116.28,103.53]" --scale_values="[58.395,57.12,57.375]" --data_type FP16
Subtraction of the mean and division by the standard deviation will be built directly into the processing graph and the inference will be run using FP16. After executing, you should see something like the following, which contains all explicit and implicit parameters such as the path to the model, input shape, precision, mean and scale values, conversion parameters, and many more:
Model Optimizer arguments:
Common parameters:
- Path to the Input Model: /home/adrian/repos/openvino_notebooks/notebooks/102-pytorch-onnx-to-openvino/fastseg.onnx
- Path for generated IR: /home/adrian/repos/openvino_notebooks/notebooks/102-pytorch-onnx-to-openvino/
- IR output name: fastseg
- Log level: ERROR
- Batch: Not specified, inherited from the model
- Input layers: Not specified, inherited from the model
- Output layers: Not specified, inherited from the model
- Input shapes: [1,3,512,1024]
- Source layout: Not specified
- Target layout: Not specified
- Layout: Not specified
- Mean values: [123.675,116.28,103.53]
- Scale values: [58.395,57.12,57.375]
- Scale factor: Not specified
- Precision of IR: FP16
- Enable fusing: True
- User transformations: Not specified
- Reverse input channels: False
- Enable IR generation for fixed input shape: False
- Use the transformations config file: None
Advanced parameters:
- Force the usage of legacy Frontend of Model Optimizer for model conversion into IR: False
- Force the usage of new Frontend of Model Optimizer for model conversion into IR: False
OpenVINO runtime found in: /home/adrian/repos/openvino_notebooks/venv/lib/python3.9/site-packages/openvino
OpenVINO runtime version: 2022.1.0-7019-cdb9bec7210-releases/2022/1
Model Optimizer version: 2022.1.0-7019-cdb9bec7210-releases/2022/1
[ SUCCESS ] Generated IR version 11 model.
[ SUCCESS ] XML file: /home/adrian/repos/openvino_notebooks/notebooks/102-pytorch-onnx-to-openvino/fastseg.xml
[ SUCCESS ] BIN file: /home/adrian/repos/openvino_notebooks/notebooks/102-pytorch-onnx-to-openvino/fastseg.bin
[ SUCCESS ] Total execution time: 0.55 seconds.
[ SUCCESS ] Memory consumed: 112 MB.
[ INFO ] The model was converted to IR v11, the latest model format that corresponds to the source DL framework input/output format. While IR v11 is backwards compatible with OpenVINO Inference Engine API v1.0, please use API v2.0 (as of 2022.1) to take advantage of the latest improvements in IR v11.
Find more information about API v2.0 and IR v11 at https://docs.openvino.ai
The word SUCCESS almost at the end denotes that everything was successfully converted. You obtained IR, which comprises two files: .xml and .bin. You are now ready to put this network into OpenVINO™ Runtime and perform the inference.
import cv2
import numpy as np
from openvino.runtime import Coreimage_filename = "data/street.jpg"
image = cv2.cvtColor(cv2.imread(image_filename), cv2.COLOR_BGR2RGB)resized_image = cv2.resize(image, (1024, 512))
input_image = np.expand_dims(np.transpose(resized_image, (2, 0, 1)), 0)
core = Core()
model_ir = core.read_model(model="fastseg.xml")
compiled_model_ir = core.compile_model(model=model_ir, device_name="CPU")
output_layer_ir = compiled_model_ir.output(0)
res_ir = compiled_model_ir([input_image])[output_layer_ir]
result_mask_ir = np.squeeze(np.argmax(res_ir, axis=1)).astype(np.uint8)
It works! The street below is segmented. You can try it yourself with this demo.

Alternatively, you can use this tool to find the best way to download and install the OpenVINO™ Toolkit regarding your environment.
Resources