Here we’ll show you how to train our CycleGAN to translate horses to zebras and zebras to horses.
Introduction
In this series of articles, we’ll present a Mobile Image-to-Image Translation system based on a Cycle-Consistent Adversarial Networks (CycleGAN). We’ll build a CycleGAN that can perform unpaired image-to-image translation, as well as show you some entertaining yet academically deep examples. We’ll also discuss how such a trained network, built with TensorFlow and Keras, can be converted to TensorFlow Lite and used as an app on mobile devices.
We assume that you are familiar with the concepts of Deep Learning, as well as with Jupyter Notebooks and TensorFlow. You are welcome to download the project code.
In the previous article, we implemented a CycleGAN from scratch. In this article, we’ll train and test the network on the horse2zebra dataset and evaluate its performance.
Training CycleGAN
Time to train our CycleGAN to perform some entertaining translations, such as horses to zebras and vice versa. We’ll start by setting a checkpoint path to save the best model:
checkpoint_path = "./checkpoints/train"
ckpt = tf.train.Checkpoint(generator_g=generator_g,
generator_f=generator_f,
discriminator_x=discriminator_x,
discriminator_y=discriminator_y,
generator_g_optimizer=generator_g_optimizer,
generator_f_optimizer=generator_f_optimizer,
discriminator_x_optimizer=discriminator_x_optimizer,
discriminator_y_optimizer=discriminator_y_optimizer)
ckpt_manager = tf.train.CheckpointManager(ckpt, checkpoint_path, max_to_keep=5)
if ckpt_manager.latest_checkpoint:
ckpt.restore(ckpt_manager.latest_checkpoint)
print ('Latest checkpoint restored!!')
For starters, we’ll train over 20 epochs and see if that is enough for acceptable results. Depending on the obtained results, we might need to increase the number of epochs. Even if your training results appear to be good, prediction may still be less accurate. Hence, 80 to 100 epochs will more likely get you perfect translation, however this will take more than 3 days of training unless you are using a system with very high specifications or paid cloud-based computing services such as AWS or Microsoft Azure.
EPOCHS = 20
def generate_images(model, test_input):
prediction = model(test_input)
plt.figure(figsize=(12, 12))
display_list = [test_input[0], prediction[0]]
title = ['Input Image', 'Predicted Image']
for i in range(2):
plt.subplot(1, 2, i+1)
plt.title(title[i])
plt.imshow(display_list[i] * 0.5 + 0.5)
plt.axis('off')
plt.show()
def train_step(real_x, real_y):
with tf.GradientTape(persistent=True) as tape:
fake_y = generator_g(real_x, training=True)
cycled_x = generator_f(fake_y, training=True)
fake_x = generator_f(real_y, training=True)
cycled_y = generator_g(fake_x, training=True)
same_x = generator_f(real_x, training=True)
same_y = generator_g(real_y, training=True)
disc_real_x = discriminator_x(real_x, training=True)
disc_real_y = discriminator_y(real_y, training=True)
disc_fake_x = discriminator_x(fake_x, training=True)
disc_fake_y = discriminator_y(fake_y, training=True)
gen_g_loss = generator_loss(disc_fake_y)
gen_f_loss = generator_loss(disc_fake_x)
total_cycle_loss = calc_cycle_loss(real_x, cycled_x) + calc_cycle_loss(real_y, cycled_y)
total_gen_g_loss = gen_g_loss + total_cycle_loss + identity_loss(real_y, same_y)
total_gen_f_loss = gen_f_loss + total_cycle_loss + identity_loss(real_x, same_x)
disc_x_loss = discriminator_loss(disc_real_x, disc_fake_x)
disc_y_loss = discriminator_loss(disc_real_y, disc_fake_y)
generator_g_gradients = tape.gradient(total_gen_g_loss,
generator_g.trainable_variables)
generator_f_gradients = tape.gradient(total_gen_f_loss,
generator_f.trainable_variables)
discriminator_x_gradients = tape.gradient(disc_x_loss,
discriminator_x.trainable_variables)
discriminator_y_gradients = tape.gradient(disc_y_loss,
discriminator_y.trainable_variables)
generator_g_optimizer.apply_gradients(zip(generator_g_gradients,
generator_g.trainable_variables))
generator_f_optimizer.apply_gradients(zip(generator_f_gradients,
generator_f.trainable_variables))
discriminator_x_optimizer.apply_gradients(zip(discriminator_x_gradients,
discriminator_x.trainable_variables))
discriminator_y_optimizer.apply_gradients(zip(discriminator_y_gradients,
discriminator_y.trainable_variables))
The training loop above does the following:
- Gets predictions
- Calculates the loss
- Calculates the gradients using backpropagation
- Applies the gradients to the optimizer
During the training, the network will select a random image from the training set and display it along with its translated version to let us visualize how the performance changes after every epoch, as shown in the figure below.
for epoch in range(EPOCHS):
start = time.time()
n = 0
for image_x, image_y in tf.data.Dataset.zip((train_horses, train_zebras)):
train_step(image_x, image_y)
if n % 10 == 0:
print ('.', end='')
n += 1
clear_output(wait=True)
generate_images(generator_g, sample_horse)
if (epoch + 1) % 5 == 0:
ckpt_save_path = ckpt_manager.save()
print ('Saving checkpoint for epoch {} at {}'.format(epoch+1,
ckpt_save_path))
print ('Time taken for epoch {} is {} sec\n'.format(epoch + 1,
time.time()-start))
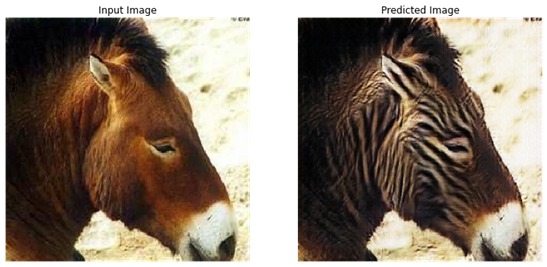
Evaluating CycleGAN
Once the CycleGAN has been trained, we can start feeding it new images and evaluating its performance in translating horses to zebras and vice versa.
Let’s test our trained CycleGAN on images from the dataset and visualize its generalization power. We’ll use the generate_images
function, which will pick up some images, pass them through the trained network, and display the translation results.
def generate_images(model, test_input):
prediction = model(test_input)
plt.figure(figsize=(12, 12))
display_list = [test_input[0], prediction[0]]
title = ['Input Image', 'Predicted Image']
for i in range(2):
plt.subplot(1, 2, i+1)
plt.title(title[i])
plt.imshow(display_list[i] * 0.5 + 0.5)
plt.axis('off')
plt.show()
Now, you can choose any test image and visualize the translation result:
for inp in test_horses.take(5):
generate_images(generator_g, inp)
Here are some examples obtained after the network had been trained over only 20 epochs. The results are quite good for such a short training. You can improve them by adding more epochs.




Season Transfer CycleGAN
We can use the network we’ve designed for different tasks, such as day to night transfer or season transfer. In order to train our network for season transfer, all we need to do is change the training dataset to summer2winter.
We trained our network on the above dataset for 80 epochs. Have a look at the results.

Next Steps
In this article, we trained a CycleGAN with a U-Net-based generator. In the next article, we’ll show you how to implement a residual-based generator and train the resulting CycleGAN on a medical dataset. Stay tuned!
Dr. Helwan is a machine learning and medical image analysis enthusiast.
His research interests include but not limited to Machine and deep learning in medicine, Medical computational intelligence, Biomedical image processing, and Biomedical engineering and systems.